Identification of patients with poor prognosis might be further improved by radiomics. Radiography uses ionizing radiation (i.e. X-rays) and non-ionizing radiation (i.e. ultrasound, MRI) to view the internal form of an object. By using artificial intelligence, radiomics technology extracts a large number of features from radiographic images. In these large datasets, machine learning is employed to search for distinct cancer characteristics which a human observer may fail to recognize. Radiomics analysis of medical scans provides quantifiable features of tumor characteristics such as intensity, shape, volume, texture, and intra-and inter-lesion heterogeneity. The aim of this project is to identify and validate radiomics features, both at patient- and cancer level, that predict treatment response and to compare these results to currently used prognostic markers.
For scientific information about this project, please contact Ronald Boellaard: r.boellaard@amsterdamumc.nl
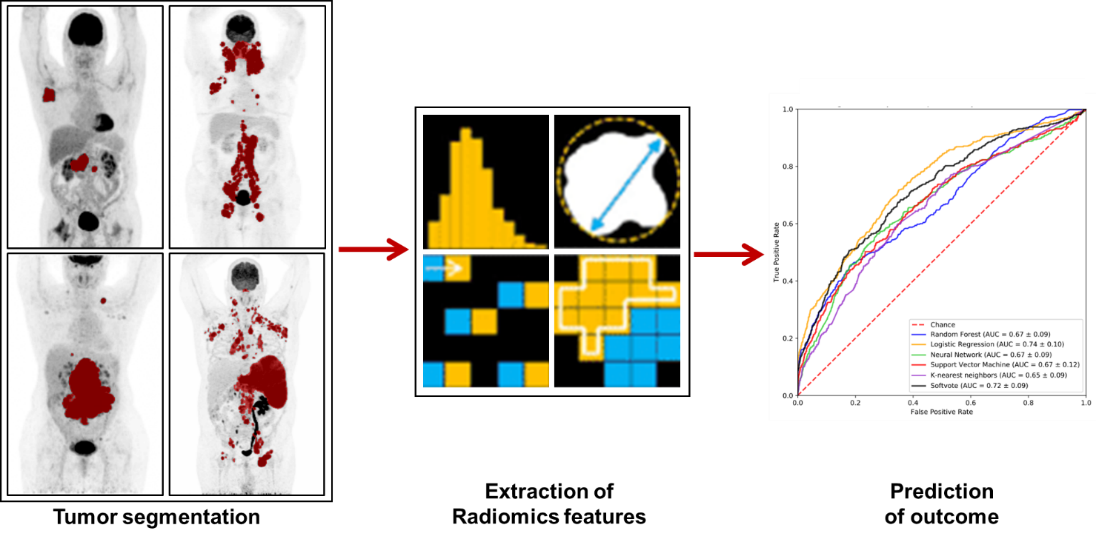
Radiomics pipeline – Left: Metabolic active cancer cells in the patient are captured by positron emission tomography (PET). Middle: Automated processing and analysis of radiographic medical images by radiomics and machine learning. Right: Modeling and prediction of disease outcome.
Researchers involved
PhD students/Research assistent: J. Eertink, S. Wiegers