Involving almost 40 Dutch hospitals, ICUdata.nl – then known as Covid Predict – was born out of the necessity to gather as much information as possible, as quickly as possible, about treatment of the new disease. Amsterdam UMC’s Paul Elbers tells about where ICUdata is going next: “The ICUdata project is quite ambitious. It is trying to combine all the data of all intensive care patients in the Netherlands that is routinely collected through the devices used for monitoring and life support.” This does not only go way beyond the project’s initial focus on treating COVID patients, it is also scaled retroactively: “Basically, the idea is to share data of all ICU patients. So not only COVID patients,” says Elbers. “And for datasets that go back at least 10 years in time.”
Analysing large sets of ICU data has an enormous amount of potential uses within ICU departments. “When we need to know how to best mechanically ventilate patients with severe pneumonia. Or we want to know which patients would benefit most from being given blood transfusions after major trauma. Or which patients would depend on which dose of antibiotics. Or which patients would benefit from vasopressors after cardiac arrests. Or... I mean, there are literally thousands of questions that can be answered by these datasets. And the larger the dataset, the better,” says Elbers.
Bringing AI to the bedside
While ICUdata.nl is concerned with the collection and sharing of the data itself, Elbers is also involved with what happens after. Within Amsterdam UMC’s department for Intensive Care Medicine, he co-chairs the Laboratory of Critical Care Computational Intelligence. Its goal, he says, is “using the insights gained from ICU data to build models using advanced statistical techniques such as machine learning and AI, and then bring those models back to the bedside.” Doctors and nurses can then get real-time advice when treating patients.
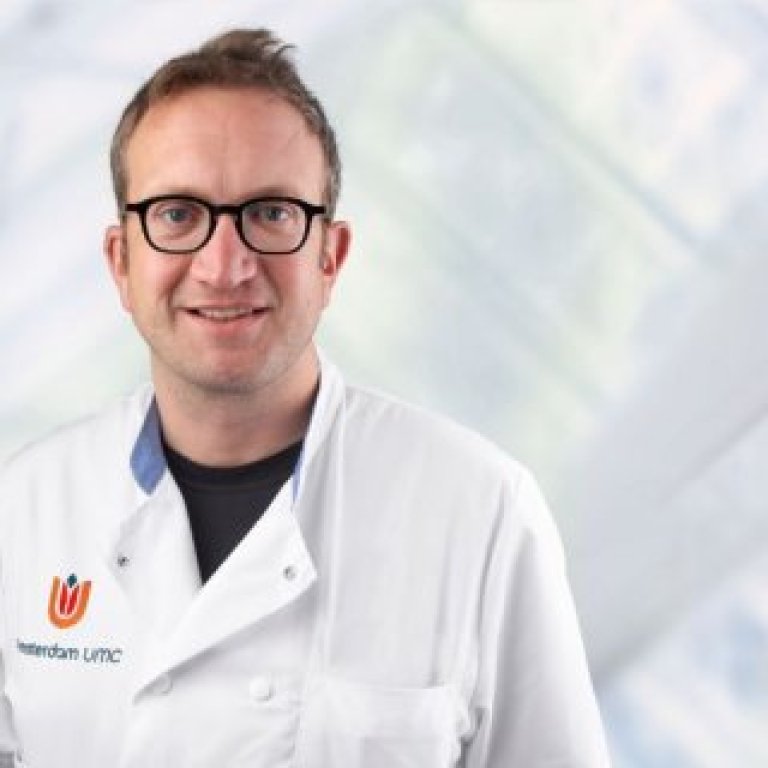
At Amsterdam UMC, this is already happening, using the results of analysing the hospital’s own data. Elbers names two examples of where models have been deployed successfully: “The first is an algorithm that helps us in deciding which patients should get which dose of antibiotic – this has been successfully implemented over the last three years. The other one is a collaboration with Pacmed– because they are our long-term partner for AI – where we have developed a readmission model. In a situation where our intensivist considers discharging a patient to the regular ward, we want to get a prediction on how likely it is that the patient will return to needing intensive care treatment, so that you might want to wait with discharging them. Reversely, if you’d consider keeping a patient in intensive care, and the algorithm says that it’s already okay to discharge them to the regular ward, then you might be influenced to do that sooner, and that would, of course, save a lot of time and money. This has now been implemented in our ICU as of last month, and is going to be tested in a trial soon. So those are two examples of how this is already happening right now: AI at the bedside.” The more data you use for models such as these, the more powerful and reliable they get and the more potential there is for new applications, so the ICUdata project can have a huge impact.
The great potential of data sharing
Like so many recent developments in healthcare, this one was given a kickstart by the coronavirus crisis. “It became painfully obvious that there was no structure in place for ICUs to share data,” Elbers says. “And that this needed to change very quickly. So we started collecting [COVID-related] data from the hospitals at record speeds. About 38 hospitals shared their data within a timeframe of two months.” While respecting all the usual regulatory and privacy protection concerns including the GDPR, other, more bureaucratic obstacles were addressed more efficiently than might have been the case before. “People were in a can-do mode,” says Elbers. “Because everyone recognised that this just needed to be solved, no matter what.”
And once the process of data sharing had started, its potential became even clearer. “It enabled us to show what our laboratory was capable of, which is combining these data in a meaningful way and producing results from it,” says Elbers.
The project ICUdata caught the attention of Zorgverzekeraars Nederland, an umbrella organisation of major Dutch health insurance companies. “They recognised our efforts and said to us and the Dutch Society for Intensive Care Medicine, can you not do that for regular ICU patients?” With the aim to gain further insights, improve treatments and efficiency, and not least to be prepared for a potential next pandemic, ICUdata was given a subsidy of €2 million.
Smart Health Amsterdam
Amsterdam UMC is one of Smart Health Amsterdam’s partners. Amsterdam UMC is also a partner in AI Technology for People; a member of the Amsterdam Economic Board’s Network Council and involved with building our regional Health Data Infrastructure.
Source: read the original article by Kim van Os at Smart Health Amsterdam.
Photography: Amsterdam UMC and Anita Edridge (portrait)